Perception classification of federal public servants regarding acts of corruption using machine learning algorithms
DOI:
https://doi.org/10.47456/bjpe.v9i4.42073Keywords:
Data Mining, Corruption, 5W2H, Federal Public ServiceAbstract
Computational techniques have proven useful in the fight against corruption in the public sector, enabling the early detection of suspicious activities. The aim of this study was to compare machine learning algorithms in the context of observing acts of corruption in the Public Service. In this regard, data extracted from a survey conducted by the World Bank in 2021 on the topic of Ethics and Corruption in the Public Service were analyzed, involving approximately 22.000 respondents. The development of models aimed at promoting transparency and integrity in the Brazilian public service is proposed. The results demonstrated the feasibility of using machine learning techniques, with Logistic Regression proving to be the best option for the studied scenario, with an accuracy of 82%. The developed model and generated analysis can be used to assist in the identification of suspicious corruption activities in the public sector, contributing to early detection and prevention of illegal practices. The results also highlight the importance of developing public policies to promote ethics and integrity in public service, as well as the role of advanced technologies in improving governance and society's trust in public institutions.
Downloads
References
Adam, I. & Fazekas, M. (2021). Are emerging technologies helping win the fight against corruption? A review of the state of evidence. Information Economics and Policy, 57. https://doi.org/10.1016/j.infoecopol.2021.100950 DOI: https://doi.org/10.1016/j.infoecopol.2021.100950
Aggarwal, C. C. (2015). Data mining: the textbook (Vol. 1). New York: springer. DOI: https://doi.org/10.1007/978-3-319-14142-8_1
Breiman, L. (2001). Random forests. Machine learning, 45. https://doi.org/10.1023/A:1010933404324 DOI: https://doi.org/10.1023/A:1010933404324
Ashforth, B. E., Gioia, D. A., Robinson, S. L., & Trevino, L. K. (2008). Re-viewing organizational corruption. Academy of Management review, 33(3). https://doi.org/10.5465/amr.2008.32465714 DOI: https://doi.org/10.5465/amr.2008.32465714
Castro, L. N. D., & Ferrari, D. G. (2016). Introdução à mineração de dados: conceitos básicos, algoritmos e aplicações. São Paulo: Saraiva, 5.
Chen, S. H. & Liao, C. C. (2011). Are foreign banks more profitable than domestic banks? Home-and host-country effects of banking market structure, governance, and supervision. Journal of Banking & Finance, 35(4). https://doi.org/10.1016/j.jbusres.2022.03.032 DOI: https://doi.org/10.1016/j.jbankfin.2010.11.006
Fawcett, T. (2006). An introduction to ROC analysis. Pattern recognition letters, 27(8). https://doi.org/10.1016/j.patrec.2005.10.010 DOI: https://doi.org/10.1016/j.patrec.2005.10.010
Fernandes, A. A. T., Figueiredo Filho, D. B., Rocha, E. C. D., & Nascimento, W. D. S. (2021). Leia este artigo se você quiser aprender regressão logística. Revista de Sociologia e Política, 28. https://doi.org/10.1590/1678-987320287406en DOI: https://doi.org/10.1590/1678-987320287406en
Gonzalez, L. D. A. (2018). Regressão logística e suas aplicações. Recuperado de https://monografias.ufma.br/jspui/bitstream/123456789/3572/1/LEANDRO-GONZALEZ.pdf
de Blasio, G., D'Ignazio, A., & Letta, M. (2022). Gotham city. Predicting ‘corrupted’ municipalities with machine learning. Technological Forecasting and Social Change, 184. https://doi.org/10.1016/j.techfore.2022.122016 DOI: https://doi.org/10.1016/j.techfore.2022.122016
Domashova, J. & Politova, A. (2021). The Corruption Perception Index: analysis of dependence on socio-economic indicators. Procedia Computer Science, 190. https://doi.org/10.1016/j.procs.2021.06.024 DOI: https://doi.org/10.1016/j.procs.2021.06.024
Fayyad, U., Piatetsky-Shapiro, G., & Smyth, P. (1996). From data mining to knowledge discovery in databases. AI magazine, 17(3). https://doi.org/10.1609/aimag.v17i3.1230
Gamarra, C., Guerrero, J. M., & Montero, E. (2016). A knowledge discovery in databases approach for industrial microgrid planning. Renewable and Sustainable Energy Reviews, 60. https://doi.org/10.1016/j.rser.2016.01.091 DOI: https://doi.org/10.1016/j.rser.2016.01.091
Gehrke, G., Borba, J. A., & Ferreira, D. D. M. (2017). A repercussão da corrupção brasileira na mídia: uma análise comparada das revistas Der Spiegel, L ‘Obs, The Economist, Time e Veja. Revista de Administração Pública, 5. http://dx.doi.org/10.1590/0034-7612158681 DOI: https://doi.org/10.1590/0034-7612158681
Géron, A. (2019). Mãos à Obra: Aprendizado de Máquina com Scikit-Learn & TensorFlow. Alta Books.
Goutte, S., Péran, T., & Porcher, T. (2022). Corruption, economy and governance in Central Africa: An analysis of public and regional drivers of corruption. Finance Research Letters, 44. https://dx.doi.org/10.2139/ssrn.3808716 DOI: https://doi.org/10.1016/j.frl.2021.102086
Jackson, D. & Köbis, N. (2018). Anti-corruption through a social norms lens. U4 Issue, 7. Recuperado de https://www.u4.no/publications/anti-corruption-through-a-social-norms-lens#conclusion-a-social-norms-approach-to-anti-corruption
James, G., Witten, D., Hastie, T., Tibshirani, R., & Taylor, J. (2023). An Introduction to Statistical Learning with Applications in Python. Springer. DOI: https://doi.org/10.1007/978-3-031-38747-0
Jancsics, D. (2019). Corruption as resource transfer: An interdisciplinary synthesis. Public Administration Review, 79(4). https://doi.org/10.1111/puar.13024 DOI: https://doi.org/10.1111/puar.13024
Jeong, D., Shenoy, A., & Zimmermann, L. V. (2023). De Jure versus De Facto transparency: Corruption in local public office in India. Journal of Public Economics, 221. https://doi.org/10.1016/j.jpubeco.2023.104855 DOI: https://doi.org/10.1016/j.jpubeco.2023.104855
Li, J., Chen, W. H., Xu, Q., Shah, N., Kohler, J. C., & Mackey, T. K. (2020). Detection of self-reported experiences with corruption on twitter using unsupervised machine learning. Social Sciences & Humanities Open, 2(1). https://doi.org/10.1016/j.ssaho.2020.100060 DOI: https://doi.org/10.1016/j.ssaho.2020.100060
Lima, M. S. M. & Delen, D. (2020). Predicting and explaining corruption across countries: A machine learning approach. Government Information Quarterly, 37(1). https://doi.org/10.1016/j.giq.2019.101407 DOI: https://doi.org/10.1016/j.giq.2019.101407
Lino, A. F., Azevedo, R. R., de., Aquino, A. C. B., de., & Steccolini, I. (2022). Fighting or supporting corruption? The role of public sector audit organizations in Brazil. Critical Perspectives on Accounting, 83. https://doi.org/10.1016/j.cpa.2021.102384 DOI: https://doi.org/10.1016/j.cpa.2021.102384
Macedo, S. V. & Valadares, J. L. (2021). Corrupção: reflexões epistemológicas e contribuições para o campo de públicas. Organizações & Sociedade, 28. https://doi.org/10.1590/1984-92302021v28n9607PT DOI: https://doi.org/10.1590/1984-92302021v28n9607pt
Mitchell, T. M. (1997). Machine learning. (Vol. 1). New York: McGraw-hill.
Pan, Z., Wang, Y., & Pan, Y. (2020). A new locally adaptive k-nearest neighbor algorithm based on discrimination class. Knowledge-Based Systems, 204.
https://doi.org/10.1016/j.knosys.2020.106185 DOI: https://doi.org/10.1016/j.knosys.2020.106185
Raschka, S. (2015). Python machine learning. Packt publishing ltd.
Speiser, J. L., Miller, M. E., Tooze, J., & Ip, E. (2019). A comparison of random forest variable selection methods for classification prediction modeling. Expert systems with applications, 134. https://doi.org/10.1016/j.eswa.2019.05.028 DOI: https://doi.org/10.1016/j.eswa.2019.05.028
Transparência Internacional. (2022). Índice de Percepção da Corrupção 2022. Recuperado de https://www.transparency.org/en/cpi/2022/index/brasil
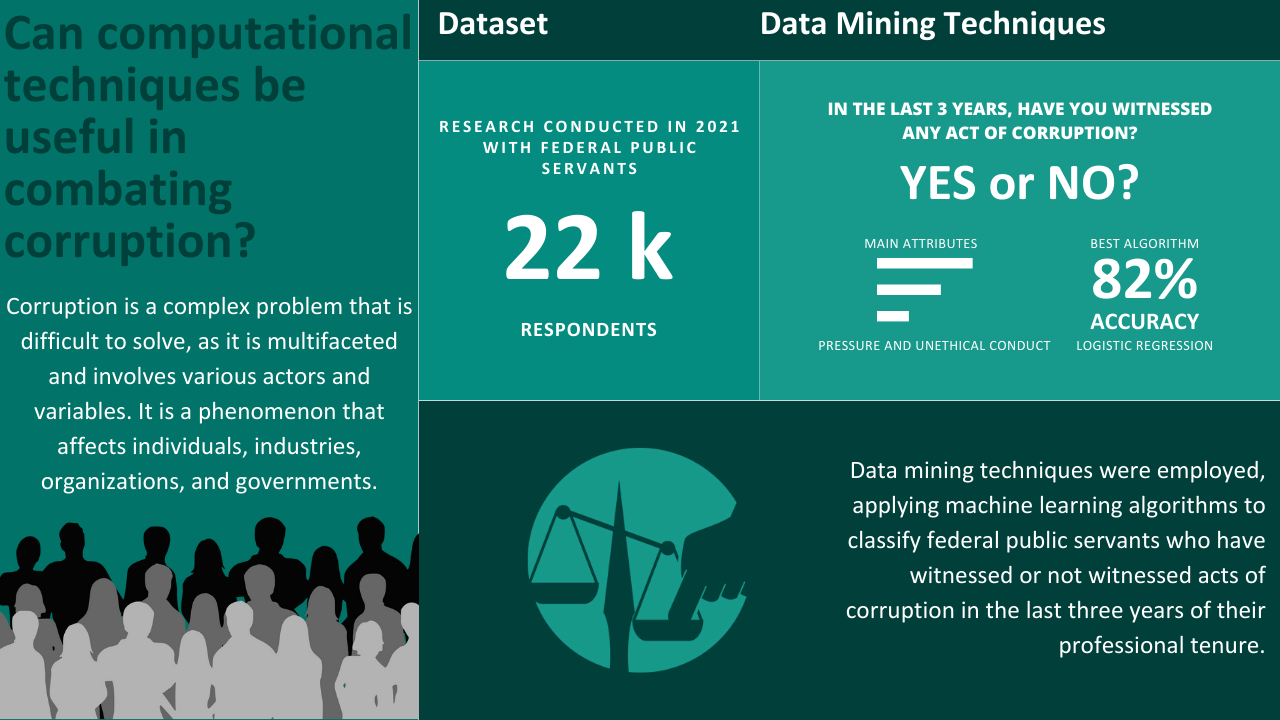
Downloads
Published
How to Cite
Issue
Section
License
Copyright (c) 2023 Brazilian Journal of Production Engineering

This work is licensed under a Creative Commons Attribution-NonCommercial-ShareAlike 4.0 International License.
