Use of machine learning models to classify user satisfaction at airports in Brazil
DOI:
https://doi.org/10.47456/bjpe.v10i2.44374Keywords:
Airports, Machine Learning, Satisfaction, ClassificationAbstract
This article describes the application of machine learning (ML) techniques using user satisfaction survey data at various airports in Brazil to classify them according to satisfaction. The K-Nearest Neighbors (KNN), Naïve Bayes, Decision Tree, and Random Forest methods were used to classify user satisfaction, and linear regression for data imputation, using the dataset from 2017 to 2022 as a training set. The data was pre-processed and cleaned. The dataset from 2017 to 2022 was used to train the model, while the most recent dataset from 2023 was used as the test set. After classification, the hyperparameter technique was applied to improve the results of the metrics. The machine learning models showed satisfactory results in classifying users. Additionally, the research revealed the main factors that affect customer satisfaction at airports, highlighting airport acoustic comfort, restroom availability, and the quantity and quality of commercial establishments as the most influential.
Downloads
References
Alpaydin, E. (2010). Introduction to Machine Learning (2nd ed.). Cambridge, MA: MIT Press.
Brasil. (2023). Portal de Dados Abertos: Indicadores que avaliam a satisfação do passageiro com os processos e serviços aeroportuários a ele oferecidos, coletados nos aeroportos durante a pesquisa (20 aeroportos principais). Recuperado de https://dados.gov.br/dados/conjuntos-dados/pesquisa-de-satisfacao-do-passageiro-em-aeroportos. Acesso em: 20/09/2023.
Breiman, L. (2001). Random Forests. Machine Learning, 45, 5-32. https://doi.org/10.1023/A:1010933404324 DOI: https://doi.org/10.1023/A:1010933404324
Costa Filho, S. V. S., Arce, J. E., Montaño, R. N. R., & Pelissari, A. L. (2019). Configuração de algoritmos de aprendizado de máquina na modelagem florestal: um estudo de caso na modelagem da relação hipsométrica. Ciência Florestal, 29(4), 1501-1515. https://doi.org/10.5902/1980509828392 DOI: https://doi.org/10.5902/1980509828392
Cranenburgh, S., Wang, S., Vij, A., Pereira, F., & Walker, J. (2022). Choice modelling in the age of machine learning - Discussion paper. Journal of Choice Modelling, 42. https://doi.org/10.1016/j.jocm.2021.100340 DOI: https://doi.org/10.1016/j.jocm.2021.100340
Gerón, A. (2019). Hands-on machine learning with scikit-learn, Keras, and TensorFlow. O’Reilly.
Ghate, V. & Hemalatha S. (2023). A comprehensive comparison of machine learning approaches with hyper-parameter tuning for smartphone sensor-based human activity recognition, Measurement: Sensors, Volume 30. https://doi.org/10.1016/j.measen.2023.100925 DOI: https://doi.org/10.1016/j.measen.2023.100925
Gupta, G. & Aggarwal, H. (2012). Improving customer relationship management using data mining. International Journal of Machine Learning and Computing, 2(6), 874–877. https://dx.doi.org/10.7763/IJMLC.2012.V2.256 DOI: https://doi.org/10.7763/IJMLC.2012.V2.256
Jäger, S., Allhorn, A. & Bießmann, F. (2021). A benchmark for data imputation methods. frontiers big data, 4. https://doi.org/10.3389/fdata.2021.693674 DOI: https://doi.org/10.3389/fdata.2021.693674
Kotler, P. (2019). Administração de Marketing. (15th ed). São Paulo: Prentice Hall.
Ludermir, T. B. (2021). Inteligência Artificial e Aprendizado de Máquina: estado atual e tendências. Inteligência Artificial: Estudos Avançados. 35(101), 85-94. https://doi.org/10.1590/s0103-4014.2021.35101.007 DOI: https://doi.org/10.1590/s0103-4014.2021.35101.007
Liashchynskyi, P., & Liashchynskyi, P. (2019). Grid Search, Random Search, Genetic Algorithm: A Big Comparison for NAS. arXiv. https://doi.org/10.48550/arXiv.1912.06059
Mayer-Schönberger, V. & Cukier, K. (2013). Big Data: A revolution that will transform how we live, work, and think. Boston: Houghton Mifflin Harcourt.
Monard, M. C. & Baranauskas, J. A. (2003). Conceitos sobre aprendizado de máquina. Sistemas inteligentes: Fundamentos e aplicações. Editora Manole.
Murphy, K. P. (2012). Machine learning: a probabilistic perspective. MIT Press.
Ngai, E. & Wu, Y. (2022). Machine learning in marketing: A literature review, conceptual framework, and research agenda. Journal of Business Research, 145. https://doi.org/10.1016/j.jbusres.2022.02.049 DOI: https://doi.org/10.1016/j.jbusres.2022.02.049
Oliveira, A. V. M., Oliveira, B. F., & Vassallo, M. D. (2023). Airport service quality perception and flight delays: Examining the influence of psychosituational latent traits of respondents in passenger satisfaction surveys. Research in Transportation Economics, 102. https://doi.org/10.1016/j.retrec.2023.101371 DOI: https://doi.org/10.1016/j.retrec.2023.101371
Pedregosa, F., et al. (2011). Scikit-learn: Machine Learning in Python. Journal of Machine Learning Research, 12(85), 2825-2830.
Perifanis, N. A. & Kitsios, F. (2023). Investigating the influence of artificial intelligence on business value in the digital era of strategy: A Literature review. information. 14 (2). https://doi.org/10.3390/info14020085 DOI: https://doi.org/10.3390/info14020085
Pontes Jr., A. P. & Fagundes, R. A. (2023). Aplicação de técnicas de otimização de hiperparâmetros em modelos de machine learning na tarefa de classificar bons e maus clientes. XVI CBIC 2023, Salvador. https://doi.org/10.21528/CBIC2023-141 DOI: https://doi.org/10.21528/CBIC2023-141
Reichheld, F. (2011). The ultimate question 2.0: How net promoter companies thrive in a customer-driven world. Harvard Business Review Press.
Ruiz, E., Yushimito, W.F., Aburto, L., & de la Cruz, R. (2024). Predicting passenger satisfaction in public transportation using machine learning models. Transportation Research Part A: Policy and Practice, 181. https://doi.org/10.1016/j.tra.2024.103995 DOI: https://doi.org/10.1016/j.tra.2024.103995
Sun, Z., Ying, W., Zhang, W., & Gong, S. (2024). Undersampling method based on minority class density for imbalanced data. Expert Systems with Applications, 249. https://doi.org/10.1016/j.eswa.2024.123328 DOI: https://doi.org/10.1016/j.eswa.2024.123328
Tene, O., Polonetsky, J. (2012). Big Data for All: Privacy and User Control in the Age of Analytics. Journal of Technology and Intellectual Property, 11 (5).
Wangkiat, P. & Polprasert, C. (2023). Machine learning approach to predict e-commerce customer satisfaction score, 2023 8th International Conference on Business and Industrial Research (ICBIR), Bangkok, Thailand. https://doi.org/10.1109/ICBIR57571.2023.10147542 DOI: https://doi.org/10.1109/ICBIR57571.2023.10147542
Witek, P. (2014). Quantum machine learning: what quantum computing means to data mining. Academic Press. https://doi.org/10.1016/C2013-0-19170-2 DOI: https://doi.org/10.1016/C2013-0-19170-2
Zaghloul, M., Barakat, S., & Rezk, A. (2024). Predicting E-commerce customer satisfaction: Traditional machine learning vs. deep learning approaches. Journal of Retailing and Consumer Services, 79. https://doi.org/10.1016/j.jretconser.2024.103865 DOI: https://doi.org/10.1016/j.jretconser.2024.103865
Zhao, Y., Zhu, W., Wei, P., Fang, P., Zhang, X., Yan, N., Liu, W., Zhao, H., & Wu, Q. (2022). Classification of Zambian grasslands using random forest feature importance selection during the optimal phenological period. Ecological Indicators, 135. https://doi.org/10.1016/j.ecolind.2021.108529 DOI: https://doi.org/10.1016/j.ecolind.2021.108529
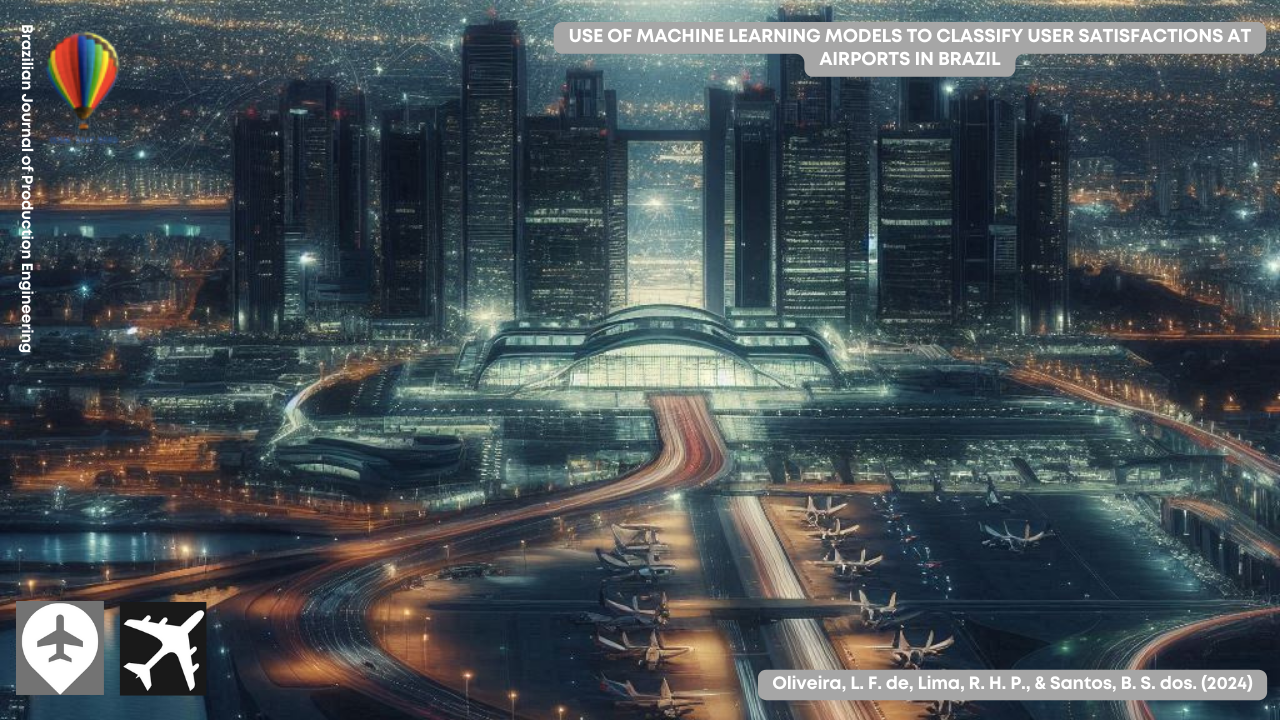
Downloads
Published
How to Cite
Issue
Section
License
Copyright (c) 2024 Brazilian Journal of Production Engineering

This work is licensed under a Creative Commons Attribution-NonCommercial-ShareAlike 4.0 International License.

Atribuição-NãoComercial-CompartilhaIgual
CC BY-NC-SA
This license lets others remix, adapt, and build upon your work non-commercially, as long as they credit you and license their new creations under the identical terms.