Drivers and enablers for the implementation of industry 4.0 technologies in performance measurement systems: a systematic review
DOI:
https://doi.org/10.47456/bjpe.v10i3.44347Keywords:
Performance Measurement Systems, Industry 4.0 Technologies, Industry 4.0Abstract
The ever-changing business landscape and evolving stakeholder requirements, coupled with the influx of complex real-time data, require more dynamic and resilient performance measurement systems (PMS). Industry 4.0 (I4.0) technologies offer significant potential in enhancing PMS. However, implementing these technologies into PMS is a complex process that faces various barriers. This article presents the findings of a systematic literature review (SLR) that explores the essential factors for implementing I4.0 technologies in PMS. Through examining 33 documents, the study highlights several key drivers, including the importance of continuous real-time monitoring of PMS for decision-makers, the potential for enhanced agility, and the prospects for improved productivity and efficiency. Additionally, the research identifies significant enablers, such as financial capacity for investment and the availability of a qualified workforce. Therefore, this study contributes to a better understanding of the drivers and enablers that support the adoption of I4.0 technologies in PMS.
Downloads
References
Ante, G., Facchini, F., Mossa, G., & Digiesi, S. (2018). Developing a key performance indicators tree for lean and smart production systems. IFAC-PapersOnLine, 51(11), 13–18. https://doi.org/10.1016/j.ifacol.2018.08.227
Assandre, J., & Martins, R. (2023). Analysis of Scientific Production on the use of Big Data Analytics in Performance Measurement Systems. IEEE Latin America Transactions, 21(3), 367–380.
Bauters, K., Cottyn, J., Claeys, D., Slembrouck, M., Veelaert, P., & van Landeghem, H. (2018). Automated work cycle classification and performance measurement for manual work stations. Robotics and Computer-Integrated Manufacturing, 51(December 2017), 139–157. https://doi.org/10.1016/j.rcim.2017.12.001
Bhadani, K., Asbjörnsson, G., Hulthén, E., & Evertsson, M. (2020). Development and implementation of key performance indicators for aggregate production using dynamic simulation. Minerals Engineering, 145(August 2019). https://doi.org/10.1016/j.mineng.2019.106065
Biolchini, J. A., Calmon, J., Mian, P. G., Natali, A. C. C., Conte, T. U., & Travassos, G. H. (2007). Scientific research ontology to support systematic review in software engineering. Advanced Engineering Informatics, 21(2), 133–151. https://doi.org/10.1016/j.aei.2006.11.006
Bititci, U., Garengo, P., Dörfler, V., & Nudurupati, S. (2012). Performance measurement: challenges for tomorrow. International Journal of Management Reviews, 14(3), 305–327. https://doi.org/10.1111/j.1468-2370.2011.00318.x
Bourne, M., Neely, A., Mills, J., & Platts, K. (2003). Implementing performance measurement systems: a literature review. International Journal of Business Performance Management, 5(1), 1–24. https://doi.org/10.1504/IJBPM.2003.002097
Chauhan, C., & Singh, A. (2019). A review of Industry 4.0 in supply chain management studies. Journal of Manufacturing Technology Management, 31(5), 863–886. https://doi.org/10.1108/JMTM-04-2018-0105
Choi, T., & Seo, Y. (2020). A real-time physical progress measurement method for schedule performance control using vision, an ar marker and machine learning in a ship block assembly process. Sensors (Switzerland), 20(18), 1–25. https://doi.org/10.3390/s20185386
Conforto, E. C., Amaral, D. C., & Silva, S. L. Da. (2011). Roteiro para revisão bibliográfica sistemática : aplicação no desenvolvimento de produtos e gerenciamento de projetos. 8° Congresso Brasileiro de Gestão de Desenvolviemnto de Produto - CNGDP 2011, 1998, 1–12.
De Mello, R. G. S., Xavier, J. E. M., & Martins, R. A. (2015). Use of big data analytics in performance measurement systems. IIE Annual Conference and Expo 2015, July, 2352–2360. https://doi.org/10.13140/RG.2.1.4071.4083
Denyer, D., & Tranfield, D. (2009). Producing a Systematic Review. In The SAGE Handbook of Organizational Research Methods (pp. 671–689). Sage Publications Ltd. https://doi.org/10.1080/03634528709378635
Dev, N. K., Shankar, R., Gupta, R., & Dong, J. (2019). Multi-criteria evaluation of real-time key performance indicators of supply chain with consideration of big data architecture. Computers and Industrial Engineering, 128(April 2018), 1076–1087. https://doi.org/10.1016/j.cie.2018.04.012
Duarte, R., De Araújo Kluska, R., Deschamps, F., De Lima, E. P., & Van Aken, E. M. (2018). A framework to integrate performance measurement systems with data analytics. 39th International Annual Conference of the American Society for Engineering Management, ASEM 2018, 1–10.
Dweekat, A., & Al-Aomar, R. (2018). An IoT-Enabled Framework for Dynamic Supply Chain Performance Management. 2018 IEEE Technology and Engineering Management Conference (TEMSCON), 1–5. https://doi.org/10.1109/TEMSCON.2018.8488417
El Abdellaoui, M. E. A., Grimaud, F., Gianessi, P., & Delorme, X. (2019). Integrated Decision Process to Design Manufacturing Systems towards Industry 4.0. IFAC-PapersOnLine, 52(13), 1373–1378.
Fabbri, S., Silva, C., Hernandes, E., Octaviano, F., Di Thommazo, A., & Belgamo, A. (2016). Improvements in the StArt tool to better support the systematic review process. Proceedings of the 20th International Conference on Evaluation and Assessment in Software Engineering, 1–5. https://doi.org/10.1145/2915970.2916013
Eltayeb, A., Mohamed, A., & Masrom, M. B. (2021). Drivers and Barriers to Implement Industry 4.0 in Manufacturing Sectors, Systematic Literature Review. The International Journal of Innovative Research in Engineering & Multidisciplinary Physical Sciences, 9, 1-9.
Fast-Berglund, Å., & Romero, D. (2019). Strategies for Implementing Collaborative Robot Applications for the Operator 4.0. IFIP Advances in Information and Communication Technology, 566(November), 682–689. https://doi.org/10.1007/978-3-030-30000-5_83
Frederico, G. F., Garza-Reyes, J. A., Anosike, A., & Kumar, V. (2019). Supply Chain 4.0: concepts, maturity and research agenda. Supply Chain Management, 25(2), 262–282. https://doi.org/10.1108/SCM-09-2018-0339
Garcia, C. A., Caiza, G., Guizado, D., Naranjo, J. E., Ortiz, A., Ayala, P., & Garcia, M. V. (2023). Visualization of Key Performance Indicators in the Production System in the Context of Industry 4.0. IFAC-PapersOnLine, 56(2), 6582–6587. https://doi.org/10.1016/j.ifacol.2023.10.310
Ghadge, A., Er Kara, M., Moradlou, H., & Goswami, M. (2020). The impact of Industry 4.0 implementation on supply chains. Journal of Manufacturing Technology Management, 31(4), 669–686. https://doi.org/10.1108/JMTM-10-2019-0368
Giusti, F., Bevilacqua, M., Tedeschi, S., & Emmanouilidis, C. (2018). Data analytics and production efficiency evaluation on a flexible manufacturing cell. 2018 IEEE International Instrumentation and Measurement Technology Conference (I2MTC), 1–6. https://doi.org/10.1109/I2MTC.2018.8409677
Horváth, D., & Szabó, R. Z. (2019). Driving forces and barriers of Industry 4.0: Do multinational and small and medium-sized companies have equal opportunities? Technological Forecasting and Social Change, 146(March), 119–132. https://doi.org/10.1016/j.techfore.2019.05.021
Hwang, G., Lee, J., Park, J., & Chang, T. W. (2017). Developing performance measurement system for Internet of Things and smart factory environment. International Journal of Production Research, 55(9), 2590–2602. https://doi.org/10.1080/00207543.2016.1245883
Kamble, S. S., Gunasekaran, A., Ghadge, A., & Raut, R. (2020). A performance measurement system for industry 4.0 enabled smart manufacturing system in SMMEs- A review and empirical investigation. International Journal of Production Economics, 229(July), 107853. https://doi.org/10.1016/j.ijpe.2020.107853
Keele, S. (2007). Guidelines for performing systematic literature reviews in software engineering. Ver. 2.3 EBSE Technical Report, 57.
Kennerley, M., & Neely, A. (2003). Measuring performance in a changing business environment. International Journal of Operations and Production Management, 23(2), 213–229. https://doi.org/10.1108/01443570310458465
Khan, S. A., Chaabane, A., & Dweiri, F. (2020). Supply chain performance measurement systems: A qualitative review and proposed conceptual framework. International Journal of Industrial and Systems Engineering, 34(1), 43–64. https://doi.org/10.1504/IJISE.2020.104315
Kibira, D., Morris, K. C., & Kumaraguru, S. (2016). Methods and tools for performance assurance of smart manufacturing systems. Journal of Research of the National Institute of Standards and Technology, 121, 282–313. https://doi.org/10.6028/jres.121.013
Kloviene, L., & Uosyte, I. (2019). Development of performance measurement system in the context of industry 4.0: A case study. Engineering Economics, 30(4), 472–482. https://doi.org/10.5755/j01.ee.30.4.21728
Konstas, K., Chountalas, P. T., Didaskalou, E. A., & Georgakellos, D. A. (2023). A Pragmatic Framework for Data-Driven Decision-Making Process in the Energy Sector: Insights from a Wind Farm Case Study. Energies, 16(17), 1–26. https://doi.org/10.3390/en16176272
Korsen, E. B. H., & Ingvaldsen, J. A. (2021). Digitalisation and the performance measurement and management system: reinforcing empowerment. International Journal of Productivity and Performance Management. https://doi.org/10.1108/IJPPM-09-2020-0488
Leitao, P., Rodrigues, N., Ferreira, A., Pagani, A., Petrali, P., & Barbosa, J. (2019). A lightweight dynamic monitoring of operational indicators for a rapid strategical awareness. 2019 IEEE International Conference on Industrial Cyber Physical Systems (ICPS), 121–126. https://doi.org/10.1109/ICPHYS.2019.8780228
Leite, L. R. (2012). Systematic literature review on performance measurement and sustainability. American Society for Engineering Management (ASEM), 869–878.
Liao, Y., Deschamps, F., Loures, E. de F. R., & Ramos, L. F. P. (2017). Past, present and future of Industry 4.0 - a systematic literature review and research agenda proposal. International Journal of Production Research, 55(12), 3609–3629. https://doi.org/10.1080/00207543.2017.1308576
Lindegren, M. L., Lunau, M. R., Mafia, M. M. P., & Ribeiro da Silva, E. (2022). Combining Simulation and Data Analytics for OEE Improvement. International Journal of Simulation Modelling (IJSIMM), 21(1), 29–40.
Lopes, M., & Martins, R. (2021). Mapping the Impacts of Industry 4 . 0 on Performance Measurement Systems. IEEE Latin America Transactions, 19(11), 1912–1923. https://latamt.ieeer9.org/index.php/transactions/article/view/4807
Lu, Y. (2017). Industry 4.0: A survey on technologies, applications and open research issues. Journal of Industrial Information Integration, 6, 1–10. https://doi.org/10.1016/j.jii.2017.04.005
Mahmoodpour, M., Lobov, A., Lanz, M., Mäkelä, P., & Rundas, N. (2018). Role-based visualization of industrial IoT-based systems. 2018 14th IEEE/ASME International Conference on Mechatronic and Embedded Systems and Applications (MESA), 1–8. https://doi.org/10.1109/MESA.2018.8449183
Mello, R., Leite, L. R., & Martins, R. A. (2014). Is big data the next big thing in performance measurement systems? IIE Annual Conference and Expo 2014, 1837–1846.
Mello, R., & Martins, R. A. (2019). Can big data analytics enhance performance measurement systems? IEEE Engineering Management Review, 47(1), 52–57. https://doi.org/10.1109/EMR.2019.2900645
Moher, D., Liberati, A., Tetzlaff, J., Altman, D. G., Altman, D., Antes, G., Atkins, D., Barbour, V., Barrowman, N., Berlin, J. A., Clark, J., Clarke, M., Cook, D., D’Amico, R., Deeks, J. J., Devereaux, P. J., Dickersin, K., Egger, M., Ernst, E., … Tugwell, P. (2009). Preferred reporting items for systematic reviews and meta-analyses: The PRISMA statement. PLoS Medicine, 6(7). https://doi.org/10.1371/journal.pmed.1000097
Morella, P., Lambán, M. P., Royo, J., Sánchez, J. C., & Latapia, J. (2020). Development of a new kpi for the economic quantification of six big losses and its implementation in a cyber physical system. Applied Sciences (Switzerland), 10(24), 1–17. https://doi.org/10.3390/app10249154
Mörth, O., Emmanouilidis, C., Hafner, N., & Schadler, M. (2020). Cyber-physical systems for performance monitoring in production intralogistics. Computers and Industrial Engineering, 142(February), 1–10. https://doi.org/10.1016/j.cie.2020.106333
Nabil, D. H., Rahman, M. H., Chowdhury, A. H., & Menezes, B. C. (2023). Managing supply chain performance using a real time Microsoft Power BI dashboard by action design research (ADR) method. Cogent Engineering, 10(2), 1–19. https://doi.org/10.1080/23311916.2023.2257924
Nader, J., Mezher, M. A., & El-Khalil, R. (2021). Towards understanding the impact of industry 4.0 technologies on operational performance: an empirical investigation in the US and EU automotive industry. Proceedings of the International Conference on Industrial Engineering and Operations Management, 2807–2822.
Neely, A., Gregory, M., & Platts, K. (2005). Performance measurement system design : a literature review and research agenda. International Journal of Operations & Production Management, 25(12), 1228–1263. https://doi.org/10.1108/01443570510633639
Neto, A. A., Deschamps, F., Da Silva, E. R., & De Lima, E. P. (2020). Digital twins in manufacturing: An assessment of drivers, enablers and barriers to implementation. Procedia CIRP, 93, 210–215. https://doi.org/10.1016/j.procir.2020.04.131
Nudurupati, S. S., Bititci, U. S., Kumar, V., & Chan, F. T. S. (2011). State of the art literature review on performance measurement. Computers and Industrial Engineering, 60(2), 279–290. https://doi.org/10.1016/j.cie.2010.11.010
Nudurupati, Sai S., & Bititci, U. S. (2005). Implementation and impact of IT-supported performance measurement systems. In Production Planning and Control (Vol. 16, Issue 2, pp. 152–162). https://doi.org/10.1080/09537280512331333057
Nudurupati, Sai S., Tebboune, S., & Hardman, J. (2016). Contemporary performance measurement and management (PMM) in digital economies. Production Planning and Control, 27(3), 226–235. https://doi.org/10.1080/09537287.2015.1092611
Pekarčíková, M., Trebuna, P., Kliment, M., Kopec, J., Dic, M., & Kronová, J. (2023). Case Study : Testing the Overall Efficiency of Equipment in the Production Process in TX Plant Simulation Software. Management and Production Engineering Review, 14(1), 34–42. https://doi.org/10.24425/mper.2023.145364
Pettigrew, A., & Whipp, R. (1993). Managing Change for Competitive Success. In Wiley-Blackwell. Wiley-Blackwell.
Pilar Lambán, M., Morella, P., Royo, J., & Carlos Sánchez, J. (2022). Using industry 4.0 to face the challenges of predictive maintenance: A key performance indicators development in a cyber physical system. Computers and Industrial Engineering,171(July). https://doi.org/10.1016/j.cie.2022.108400
Raffoni, A., Visani, F., Bartolini, M., & Silvi, R. (2018). Business Performance Analytics: exploring the potential for Performance Management Systems. In Production Planning and Control (Vol. 29, Issue 1, pp. 51–67). https://doi.org/10.1080/09537287.2017.1381887
Raji, I. O., Shevtshenko, E., Rossi, T., & Strozzi, F. (2021). Industry 4.0 technologies as enablers of lean and agile supply chain strategies: an exploratory investigation. International Journal of Logistics Management. https://doi.org/10.1108/IJLM-04-2020-0157
Rasool, F., Greco, M., & Grimaldi, M. (2021). Digital supply chain performance metrics: a literature review. Measuring Business Excellence, January. https://doi.org/10.1108/MBE-11-2020-0147
Reinsel, D., Gantz, J., & Rydning, J. (2018). Data Age 2025: The Digitization of the World From Edge to Core. In Seagate, IDC (Issue November). https://www.seagate.com/files/www-content/our-story/trends/files/idc-seagate-dataage-whitepaper.pdf
Rezqianita, B. L., & Ardi, R. (2020). Drivers and Barriers of Industry 4.0 Adoption in Indonesian Manufacturing Industry. ACM International Conference Proceeding Series, 123–128. https://doi.org/10.1145/3400934.3400958
Saez, M., Maturana, F. P., Barton, K., & Tilbury, D. M. (2018). Real-Time Manufacturing Machine and System Performance Monitoring Using Internet of Things. IEEE Transactions on Automation Science and Engineering, 15(4), 1735–1748. https://doi.org/10.1109/TASE.2017.2784826
Samir, K., Khabbazi, M., Maffei, A., & Onori, M. (2018). Key Performance Indicators in Cyber-Physical Production Systems. Procedia CIRP, 72, 498–502.
Sandengen, O. C., Estensen, L. A., Rodseth, H., & Schjolberg, P. (2016). High Performance Manufacturing - An Innovative Contribution towards Industry 4.0. International Workshop of Advanced Manufacturing and Automation (IWAMA 2016), 14–20. https://doi.org/10.2991/iwama-16.2016.3
Sardi, A., Sorano, E., Cantino, V., & Garengo, P. (2020). Big data and performance measurement research: trends, evolution and future opportunities. Measuring Business Excellence, June 2019. https://doi.org/10.1108/MBE-06-2019-0053
Schröder, C. (2016). The Challenges of Industry 4.0 for Small and Medium-sized Enterprises. The Friedrich-Ebert-Stiftung, 28. www.fes-2017plus.de
Schwab, K. (2016). A quarta revolução industrial. In Edipro. Edipro.
Siddaway, A. P., Wood, A. M., & Hedges, L. V. (2019). How to Do a Systematic Review: A Best Practice Guide for Conducting and Reporting Narrative Reviews, Meta-Analyses, and Meta-Syntheses. Annual Review of Psychology, 70(1), 747–770. https://doi.org/10.1146/annurev-psych-010418-102803
Stentoft, J., Adsbøll Wickstrøm, K., Philipsen, K., & Haug, A. (2020). Drivers and barriers for Industry 4.0 readiness and practice: empirical evidence from small and medium-sized manufacturers. Production Planning and Control, 1–18. https://doi.org/10.1080/09537287.2020.1768318
Supekar, S. D., Graziano, D. J., Riddle, M. E., Nimbalkar, S. U., Das, S., Shehabi, A., & Cresko, J. (2019). A framework for quantifying energy and productivity benefits of smart manufacturing technologies. Procedia CIRP, 80, 699–704.
Thomé, A. M. T., Scavarda, L. F., & Scavarda, A. J. (2016). Conducting systematic literature review in operations management. Production Planning and Control, 27(5), 408–420. https://doi.org/10.1080/09537287.2015.1129464
Türkeş, M. C., Oncioiu, I., Aslam, H. D., Marin-Pantelescu, A., Topor, D. I., & Căpuşneanu, S. (2019). Drivers and barriers in using industry 4.0: A perspective of SMEs in Romania. Processes, 7(3), 1–20. https://doi.org/10.3390/pr7030153
Venuta, M., Zambetti, M., Pirola, F., Pezzotta, G., Grasseni, P., Ferrari, M., & Salvi, S. (2021). Implementation of IoT Platform’s Dashboards for the Visualisation of Dynamic KPIs: Insights from a Case Study. Working Conference on Virtual Enterprises, 517–525. https://doi.org/10.1007/978-3-030-85969-5_48
Wang, W., Zhang, Y., Gu, J., & Wang, J. (2021). A Proactive Manufacturing Resources Assignment Method Based on Production Performance Prediction for the Smart Factory. IEEE Transactions on Industrial Informatics, 18(1), 46–55. https://doi.org/10.1109/TII.2021.3073404
Zhou, J., Wang, Y., & Chua, Y. Q. (2020). Machine OEE Monitoring and Analysis for a Complex Manufacturing Environment. 2020 15th IEEE Conference on Industrial Electronics and Applications (ICIEA), 1413–1418. https://doi.org/10.1109/ICIEA48937.2020.9248351
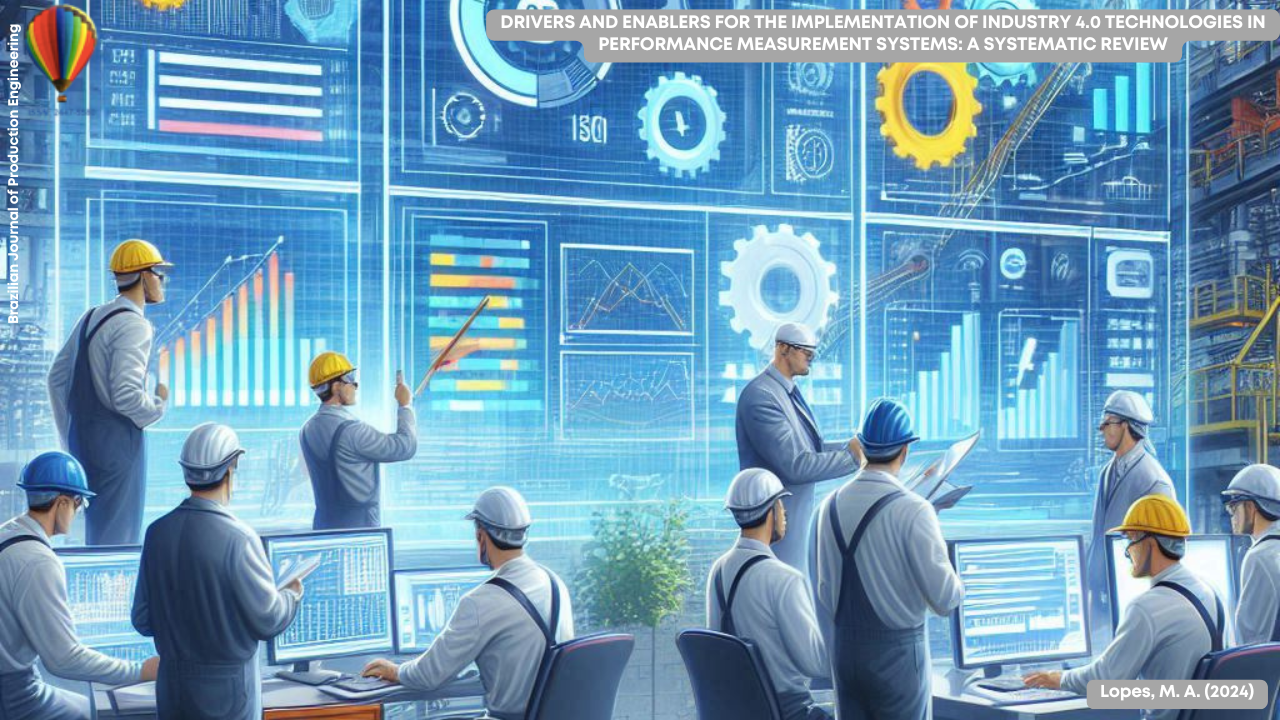
Downloads
Published
How to Cite
Issue
Section
License
Copyright (c) 2024 Brazilian Journal of Production Engineering

This work is licensed under a Creative Commons Attribution 4.0 International License.
