Transforming data into information: application of business intelligence for automating data analysis in healthcare
DOI:
https://doi.org/10.47456/bjpe.v10i3.44927Keywords:
Business IntelligenceAbstract
Context: Recent scientific advances indicate a new frontier in epidemiology: the analysis of large datasets (Big Data), where Business Intelligence tools play a fundamental role. Objective: To implement a Data Warehouse (DW) for health data analysis and test its use in analyzing mental health indicators in Espírito Santo (ES). Methods: The study was divided into four phases: 1) identification of databases and indicators; 2) data extraction, transformation, and loading; 3) creation of a Data Warehouse; and 4) analytical processing with data visualization. Results: The creation of the DW identified that R$ 53.7 million was spent on mental health-related hospitalizations in Espírito Santo, with a progressive reduction over the years. During the COVID-19 pandemic, there was an over 100% increase in deaths related to mental disorders, especially linked to alcohol and tobacco use, more common among brown-skinned individuals, males, and those aged 45 to 59 years. Conclusion: The implementation of a DW for health data analysis enabled the identification of important mental health indicators in Espírito Santo and will allow for new analyses in the context of public health in ES and Brazil.
Downloads
References
Ali, M. S., Amorim, L. D. A. F., Werneck, G. L., Bittencourt, S. A., Hartz, Z., Barreto, M. L., ... & Oliveira, C. S. (2019). Administrative data linkage in Brazil: Potentials for health technology assessment. Frontiers in Pharmacology, 10. https://doi.org/10.3389/fphar.2019.00984 DOI: https://doi.org/10.3389/fphar.2019.00984
Batko, K. & Ślęzak, A. (2022). The use of big data analytics in healthcare. Journal of Big Data, 9(1). https://doi.org/10.1186/s40537-021-00553-4 DOI: https://doi.org/10.1186/s40537-021-00553-4
Cheng, K. Y., Pazmino, S., & Schreiweis, B. (2022). ETL processes for integrating healthcare data - tools and architecture patterns. Studies in Health Technology and Informatics, 299, 151–156. https://doi.org/10.3233/SHTI220974 DOI: https://doi.org/10.3233/SHTI220974
Chiavegatto Filho, A. D. P. (2015). Uso de big data em saúde no Brasil: Perspectivas para um futuro próximo. Epidemiologia e Serviços de Saúde, 24(2), 325–332. https://doi.org/10.5123/S1679-49742015000200015 DOI: https://doi.org/10.5123/S1679-49742015000200015
Coelho Neto, G. C. & Chioro, A. (2021). Afinal, quantos Sistemas de Informação em Saúde de base nacional existem no Brasil? Cadernos de Saúde Pública, 37. https://doi.org/10.1590/0102-311X00182119 DOI: https://doi.org/10.1590/0102-311x00182119
Coelho, F. C., Araújo, W., Oliveira, M., Oliveira, L., Lima, A., & Silva, G. (2021). AlertaDengue/PySUS: Vaccine. Zenodo. https://doi.org/10.5281/zenodo.4883502
Coeli, C. M. (2022). Ciência de dados populacionais. Epidemiologia e Serviços de Saúde, 31(3). https://doi.org/10.1590/S2237-96222022000300001 DOI: https://doi.org/10.1590/s2237-96222022000300001
Cozzoli, N., Minicucci, M. F., Siciliano, C. B., Celi, L. A., & Iadanza, E. (2022). How can big data analytics be used for healthcare organization management? Literary framework and future research from a systematic review. BMC Health Services Research, 22(1). https://doi.org/10.1186/s12913-022-08167-z DOI: https://doi.org/10.1186/s12913-022-08167-z
Daniel, V. M., Pereira, G. V., & Macadar, M. A. (2014). Perspectiva Institucional dos Sistemas de Informação em Saúde em Dois Estados Brasileiros. Revista de Administração Contemporânea, 18, 650–669. https://doi.org/10.1590/1982-7849rac20141709 DOI: https://doi.org/10.1590/1982-7849rac20141709
Evangelou, E., Gill, D., Brown, M. R., Elliott, P., Tzoulaki, I., Murphy, N., ... & Holmes, M. V. (2021). Alcohol consumption in the general population is associated with structural changes in multiple organ systems. eLife, 10, e65325. https://doi.org/10.7554/eLife.65325 DOI: https://doi.org/10.7554/eLife.65325
Faria, R. J., Marques, C. D. L., Duarte, A. L. B. P., & Ribeiro, C. A. (2022). Conventional synthetic disease-modifying anti-rheumatic drugs for psoriatic arthritis: Findings and implications from a patient-centered longitudinal study in Brazil. Frontiers in Pharmacology, 13. https://doi.org/10.3389/fphar.2022.878972 DOI: https://doi.org/10.3389/fphar.2022.878972
Fernandes, F. T. & Chiavegatto Filho, A. D. P. (2019). Perspectivas do uso de mineração de dados e aprendizado de máquina em saúde e segurança no trabalho. Revista Brasileira de Saúde Ocupacional, 44. https://doi.org/10.1590/2317-6369000019418 DOI: https://doi.org/10.1590/2317-6369000019418
FIOCRUZ – Fundação Oswaldo Cruz. (2023). Plataforma de Ciência de Dados aplicada à Saúde (PCDaS). Laboratório de Informação em Saúde (Lis). Instituto de Comunicação e Informação Científica e Tecnológica em Saúde (Icict). Recuperado de https://pcdas.icict.fiocruz.br. https://doi.org/10.7303/syn25882127
Gaioti, C. S. (2024). Saúde mental no ES. Tableau.com. Recuperado de https://public.tableau.com/app/profile/cleiton.schwambach.gaioti/viz/SadeMentalnoES/HospitalizaesebitosporSadeMentalnoES?publish=yes
Heck, E. V. (2019). Big data and disruptions in business models. RAE: Revista de Administração de Empresas, 59(6), 430–432. https://doi.org/10.1590/S0034-759020190608 DOI: https://doi.org/10.1590/s0034-759020190608
Kimball, R. & Ross, M. (2013). The data warehouse toolkit: The definitive guide to dimensional modeling (3rd ed.). John Wiley & Sons, Inc.
Kupcova, I., Fauskanger, J., Cederberg, S. A., Szabo, M., Bygstad, B., & Faxvaag, A. (2023). Effects of the COVID-19 pandemic on mental health, anxiety, and depression. BMC Psychology, 11(1). https://doi.org/10.1186/s40359-023-01130-5 DOI: https://doi.org/10.1186/s40359-023-01130-5
Maior, M. da C. L. S., Osorio-de-Castro, C. G. S., & Andrade, C. L. T. de. (2020). Demografia, óbitos e indicadores de agravamento nas internações por intoxicações medicamentosas entre menores de 5 anos no Brasil. Revista Brasileira de Epidemiologia, 23. https://doi.org/10.1590/1980-549720200016 DOI: https://doi.org/10.1590/1980-549720200016
Medeiros, M. M., Maçada, A. C. G., & Hoppen, N. (2021). The role of big data stewardship and analytics as enablers of corporate performance management. RAM. Revista de Administração Mackenzie, 22(6). https://doi.org/10.1590/1678-6971/eRAMD210063 DOI: https://doi.org/10.1590/1678-6971/eramd210063
Mehta, G. & Sheron, N. (2019). No safe level of alcohol consumption – implications for global health. Journal of Hepatology, 70(4), 587–589. https://doi.org/10.1016/j.jhep.2018.12.021 DOI: https://doi.org/10.1016/j.jhep.2018.12.021
Millwood, I. Y., Walters, R. G., Fillmore, T., Cheng, Y., Lacey, B., Lu, Y., ... & Bennett, D. A. (2023). Alcohol intake and cause-specific mortality: Conventional and genetic evidence in a prospective cohort study of 512,000 adults in China. The Lancet Public Health, 8(12), e956-e967. https://doi.org/10.1016/S2468-2667(23)00217-7 DOI: https://doi.org/10.1016/S2468-2667(23)00217-7
Ognjanovic, I. (2020). Healthcare data analytics. Studies in Health Technology and Informatics, 274, 122–135. https://doi.org/10.3233/SHTI200672 DOI: https://doi.org/10.3233/SHTI200672
Oliveira, H. F. de, Sampaio, A. L. L., & Oliveira, C. A. C. P. de. (2011). DATASUS as an instrument for developing otologic public health policies. Brazilian Journal of Otorhinolaryngology, 77(3), 369–372. https://doi.org/10.1590/S1808-86942011000300016 DOI: https://doi.org/10.1590/S1808-86942011000300016
Paim, J., Travassos, C., Almeida, C., Bahia, L., & Macinko, J. (2011). The Brazilian health system: History, advances, and challenges. The Lancet, 377(9779), 1778-1797. https://doi.org/10.1016/S0140-6736(11)60054-8 DOI: https://doi.org/10.1016/S0140-6736(11)60054-8
Santomaura, D. F., Santomauro, D. F., Mantilla Herrera, A. M., Shadid, J., Zheng, P., Ashbaugh, C., ... & Ferrari, A. J. (2021). Global prevalence and burden of depressive and anxiety disorders in 204 countries and territories in 2020 due to the COVID-19 pandemic. The Lancet, 398(10312), 1700–1712. https://doi.org/10.1016/S0140-6736(21)02143-7 DOI: https://doi.org/10.1016/S0140-6736(21)02143-7
Topiwala, A., Ebmeier, K. P., Jardine, R., Nichols, T. E., Ward, E. V., & Kivimaki, M. (2022). Alcohol consumption and MRI markers of brain structure and function: Cohort study of 25,378 UK Biobank participants. NeuroImage: Clinical, 35, 103066. https://doi.org/10.1016/j.nicl.2022.103066 DOI: https://doi.org/10.1016/j.nicl.2022.103066
Viana, S. A. P., Tanaka, S. O., & Santos, T. M. (2022). Prontuário Eletrônico do Paciente em um hospital universitário: Análise dos registros de atendimento. Revista Brasileira de Enfermagem, 75. https://doi.org/10.1590/0034-7167-2022-0031
Williams, J. (2018). Data management challenges in healthcare: Information quality and effective management. Journal of Data and Information Quality, 10(1), 1–8. https://doi.org/10.1145/3183330 DOI: https://doi.org/10.1145/3190579
Woolhandler, S. & Himmelstein, D. U. (2017). Single-payer reform: The only way to fulfill the president's pledge of more coverage, better benefits, and lower costs. Annals of Internal Medicine, 166(8), 587-588. https://doi.org/10.7326/M17-0302 DOI: https://doi.org/10.7326/M17-0302
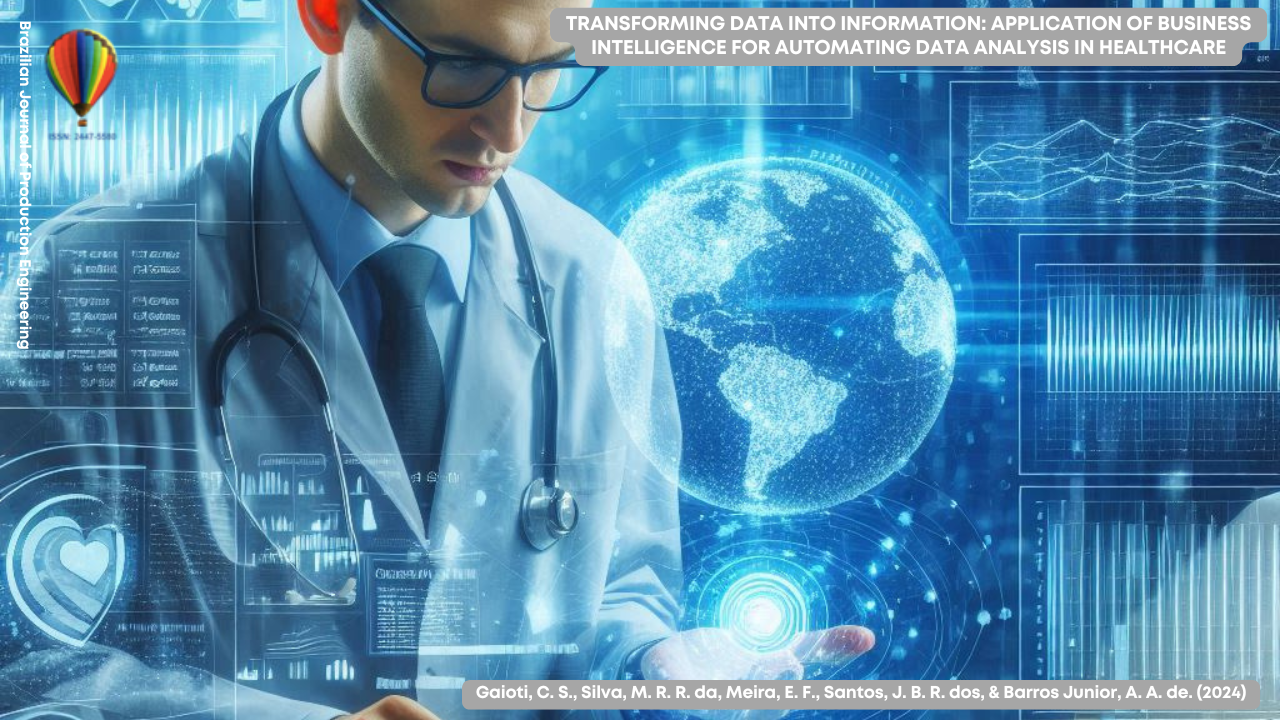
Downloads
Published
How to Cite
Issue
Section
License
Copyright (c) 2024 Brazilian Journal of Production Engineering

This work is licensed under a Creative Commons Attribution 4.0 International License.
